I work in a team that is navigating a way forward for a large, high-tech organisation to get on the right trajectory with AI. This technology is not new to them and, indeed, they have been pioneers of AI over the last couple of decades. However, their use of AI has been in very specialised areas whereas the opportunity now is to use AI much more widely across their entire global enterprise. We are now into our second year of helping them and we have unearthed a number of insights. One insight, in particular, is quite key to what we do next: the term ‘data scientist’ is being overloaded.
What do we mean? Using AI to help people make better decisions is one of the most-cited and most-pursued application areas for AI whether its in medicine, law, etc. However, data science doesn’t train us to be good psychologists or behavioural scientists or user researchers. This most important and deeply human activity – understanding a situation, knowing what you are trying to achieve, considering your options, trusting (or not) the information you have – is complex, personal and ambiguous. Extracting useful information from data is an important job of the competent data scientist. Understanding what a decision-maker is trying to achieve, the decisions they need to make, the information they need to make a good decision, etc. is not within the toolkit of any data scientist I know. And arguably shouldn’t be.
Should we, instead, look to the discipline of software engineering and user researchers / user experience research + development to cover some of this? I don’t think it does, primarily because software engineering is seeking to answer the question “how do I maximise the utility of this piece of software for this user?” rather than “what top-level outcomes is my user trying to achieve?”.
Market research and focus groups approach this question from the field of economics and behavioural science. But, yet again, the goal is different and, in this case, is: how do I best understand the latent + unmet needs of certain types of people and convince them to buy this product?
The only discipline that I have found that solely focuses on helping a person make good decisions is, unsurprisingly, decision intelligence (which builds on related disciplines such as decision analysis and decision engineering). To illustrate, consider this extract from Lorien Pratt’s upcoming book The Decision Intelligence Handbook:
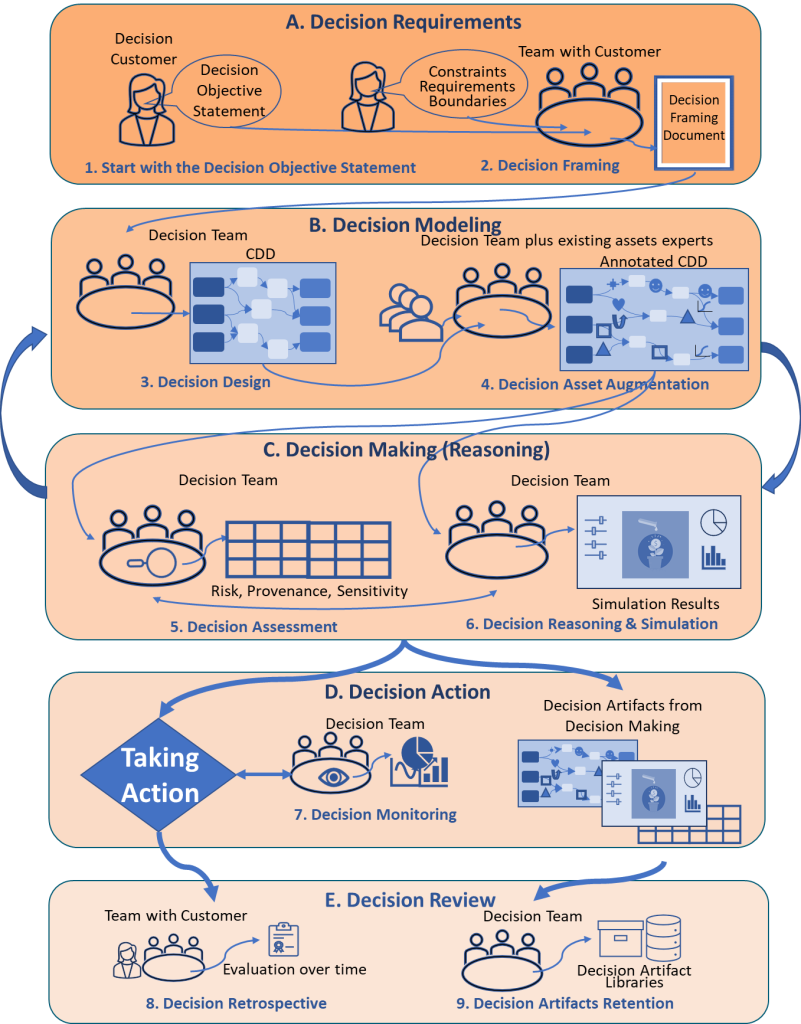
Now, we don’t need to be more too concerned on the actual steps described in Lorien’s diagram. The point here is that there is (a) considerably more to decision-making than extracting information from data, and (b) a different framing of the problem by coming at it from what outcomes someone is trying to achieve and the decisions they believe will have a direct effect on the achievement of that outcome. Whether it’s a solo decision or a team decision, the scope of enquiry that a person considers is broader than simply analysing the information that is presented to them.
By taking a more decision-centric approach to understanding where to use data and AI we stand a better chance of investing our time and resources on those things that directly supports the decisions that count towards achieving outcomes.
Decision intelligence (or, if you are adverse to that phrase, see it as a decision-centric approach to developing safe + effective AI applications), is a discipline that is rapidly entering the mainstream of business and operations management. When we come to consider where best to use data and AI we should start by understanding the outcomes we are trying to achieve and, therefore, the detailed nature of the decisions we need to make to pursue them.
To make valuable use of we need to provide clear, consistent and good quality aiming points for our data scientists and software engineers who will very much welcome the clarity and confidence that this brings.